Keynote Speakers
- Omar Ghattas (ICES - Austin, Texas, USA)
- Luc Giraud (INRIA - Bordeaux, Gironde, France)
- Bruno Schulze (LNCC - Petropolis, Rio de Janeiro, Brazil)
- Mateo Valero (BCS - Barcelona, Spain)
Omar Ghattas
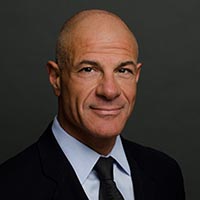
Dr. Omar Ghattas is the John A. and Katherine G. Jackson Chair in Computational Geosciences, Professor of Geological Sciences and Mechanical Engineering, and Director of the Center for Computational Geosciences in the Institute for Computational Engineering and Sciences (ICES) at The University of Texas at Austin. He is also a member of the faculty in the Computational Science, Engineering, and Mathematics (CSEM) interdisciplinary PhD program in ICES, serves as Director of the KAUST-UT Austin Academic Excellence Alliance, and holds courtesy appointments in Computer Science, Biomedical Engineering, the Institute for Geophysics, and the Texas Advanced Computing Center. Prior to coming to UT-Austin in 2005, he was a professor at Carnegie Mellon University for 16 years. He earned BS, MS, and PhD degrees from Duke University in 1984, 1986, and 1988.
Ghattas has general research interests in simulation and modeling of complex geophysical, mechanical, and biological systems on supercomputers, with specific interest in inverse problems and associated uncertainty quantification for large-scale systems. His center's current research is aimed at large-scale forward and inverse modeling of whole-earth, plate-boundary-resolving mantle convection; global seismic wave propagation; dynamics of polar ice sheets and their land, atmosphere, and ocean interactions; and subsurface flows, as well as the underlying computational, mathematical, and statistical techniques for making tractable the solution and uncertainty quantification of such complex forward and inverse problems on parallel supercomputers.
Ghattas received the ACM Gordon Bell Prize in 2003 (for Special Achievement) and again in 2015 (for Scalability), and was a finalist for the 2008, 2010, and 2012 Bell Prizes. He also received the 1998 Allen Newell Medal for Research Excellence, 2004/2005 CMU College of Engineering Outstanding Research Prize, SC02 Best Technical Paper Award, SC06 HPC Analytics Challenge Award, 2008 TeraGrid Capability Computing Challenge award, XSEDE12 Best Visualization Award, 2012 Jackson School of Geosciences Joseph C. Walter Excellence Award, and Best Poster Prize at SC09 and SC14. He has served on the editorial boards or as associate editor of 16 journals, has been co-organizer of 12 conferences and workshops and served on the scientific or program committees of 51 others, has delivered invited keynote or plenary lectures at 34 international conferences, and has been a member or chair of 28 national or international professional or governmental committees. He is a Fellow of the Society for Industrial and Applied Mathematics (SIAM).
Abstract
Scalable Algorithms for Bayesian Inference of Large-Scale Models from Large-Scale Data
Many physical systems are characterized by complex nonlinear behavior coupling multiple physical processes over a wide range of length and time scales. Mathematical and computational models of these systems often contain numerous uncertain parameters, making high-reliability predictive modeling a challenge. Rapidly expanding volumes of observational data - along with tremendous increases in HPC capability - present opportunities to reduce these uncertainties via solution of large-scale inverse problems. Bayesian inference provides a systematic framework for inferring model parameters with associated uncertainties from (possibly noisy) data and any prior information. However, solution of Bayesian inverse problems via conventional Markov chain Monte Carlo (MCMC) methods remains prohibitive for expensive models and high-dimensional parameters, as result from discretization of infinite dimensional problems with uncertain fields. Despite the large size of observational datasets, typically they can provide only sparse information on model parameters, due to ill-posedness of the inverse problem. Based on this property we design scalable Bayesian inversion algorithms that adapt to the structure of the posterior probability and exploit an effectively-reduced parameter dimension, thereby making Bayesian inference tractable for some large-scale, high-dimensional inverse problems. We discuss an inverse problem for the flow of the Antarctic ice sheet, which has been solved for as many as one million uncertain parameters at a cost (measured in forward ice sheet flow solves) that is independent of the parameter dimension, the data dimension, and the number of processor cores. This work is joint with Tobin Isaac, Noemi Petra, and Georg Stadler.
Luc Giraud
Luc Giraud joined Inria as a senior research scientist in 2009, since 2011 he leads the Inria project team HiePACS that addresses the scalability challenges in large scale numerical simulations.
He got an engineer degree in computer science and applied math at ENSEEIHT in 1988 and completed a PhD in applied math at Institut National Polyetchnique Toulouse (INPT) in 1991 on parallel chaotic relaxations. He joined CERFACS first as research scientist and became deputy project leader of the Parallel Algorithms team, before to move to INPT for a full professor position in applied math in 2005.
His research interests include high performance computing, numerical linear algebra and large scale simulations.
Abstract
Numerical resiliency in iterative linear algebra calculation
The advent of extreme scale machines will require the use of parallel resources at an unprecedented scale, probably leading to a high rate of hard faults and soft errors. Handling fully these faults at the computer system level may have a prohibitive cost. High performance computing applications that aim at exploiting all these resources will thus need to be resilient, i.e., be able to compute a correct solution in presence of faults. We focus on numerical linear algebra problems such as the solution of linear systems or eigen problems that are the innermost numerical kernels in many scientific and engineering applications and also ones of the most time consuming parts.
To address hard fault on computing core, we first present possible remedies based on recovery techniques followed by restarting strategies. In the framework of Krylov subspace linear solvers the lost entries of the iterate are interpolated using the available entries on the still alive cores to define a new initial guess before restarting the Krylov method. In particular, we consider two interpolation policies that preserve key numerical properties of well-known linear solvers.
Tackling silent data corruption (SDC) induced by soft-error is somehow more complex as we first need to better understand the impact of SDC on the numerical behavior of the solution scheme. The next step is the design of numerical criteria to possibly detect the faults that prevent to converge and eventually the design of a recovery scheme. In the context of the well-known Conjugate Gradient method we illustrate these three steps as well as preliminary results for GMRES.
Joint work with E. Agullo (Inria), A. Moreau (Inria), P. Salas Medina (Sherbrooke University), E. F. Yetkin (Inria), M. Zounon (The University of Manchester).
Bruno Schulze
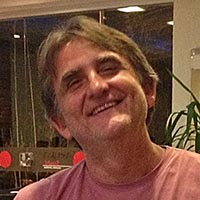
PhD in Computer Science (UNICAMP) / Fraunhofer Institute for Open Communication Systems (FOKUS (sand.). MSc in Electrical Engineering (COPPE / UFRJ). Senior Researcher / Professor, National Laboratory for Scientific Computing (LNCC). Expertise in Computing with emphasis on Architecture of Computer Systems, particularly on topics such as: middleware, cloud computing, e-science, security, scalability, virtualization, simulations, among others. Guest Editor of journal special issues on Clouds and Grids with Concurrency and Computation: Practice & Experience (1532-0626), and reviewer for journals such as IEEE Distributed Systems Online (1541-4922), Computer Networks (1389-1286), Computer Communications (0140-3664), Information and Software Technology (0950 -5849). Associated Editor for IEEE Transactions on Cloud Computing (TCC). Coordinator of Projects in different Grids, Cloud, and Multicores. Societies: ACM, IEEE-CS, SBC, SBPC, SBMAC. Currently involved in an EU / BR funded project on HPC for Energy.
\Abstract
HPC as a Service
Several studies have been carried out to check limitations of clouds in providing support to scientific applications. The major part is dedicated to the behavior of scientific applications, most of them characterized by large amounts of information processing and massive use of computational resources. In this context clouds emerge in providing additional resources, or in minimizing cost in the acquisition of new resources. The use of clouds in support to scientific applications have inherented characteristics, different from the commercial ones. The virtualization technologies, are the basic elements of clouds' infrastructure, and despite of their significant advances they still present limitations when confronted with the needs of high computational power and communication, demanded by several scientific applications. However, using virtualized resources demands a deeper understanding of the characteristics of the applications and the cloud architecture. Our group of Distributed Scientific Computing at the National Laboratory for Scientific Computing (ComCidis / LNCC) and other research groups, suggest that different virtualization layers and hardware architecture used in the cloud infrastructure, influence the performance of scientific applications. This influence leads to the concept of affinity, i.e., which group of scientific applications has a better performance associated to the virtualization layer and hardware architecture beeing used. These aspects involve: a) to reduce cloud environment limitations in support to scientific applications; b) to provide the basis for the development of new cloud scheduling algorithms; c) to assist the acquisition of new resources and cloud providers, looking for performance and resource usage optimization. The ComCiDis group is developing a set of research projects aiming to understand the relationship among: scientific applications, virtualization layers and infrastructure, based on its private development cloud platform named Neblina. The platform should enable prospecting new technologies and solutions in optimizing the use of cloud environments.
Mateo Valero
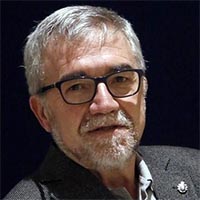
Mateo Valero obtained his Telecommunication Engineering Degree from the Technical University of Madrid (UPM) in 1974 and his Ph.D. in Telecommunications from the Technical University of Catalonia (UPC) in 1980. He is a professor in the Computer Architecture Department at UPC, in Barcelona. His research interests focuses on high performance architectures. He has published approximately 700 papers, has served in the organization of more than 300 International Conferences and he has given more than 400 invited talks. He is the director of the Barcelona Supercomputing Centre, the National Centre of Supercomputing in Spain.
Dr. Valero has been honoured with several awards. Among them, the Eckert-Mauchly Award 2007 by the IEEE and ACM; Seymour Cray Award 2015 by IEEE; Harry Goode Award 2009 by IEEE: ACM Distinguished Service Award 2012; Euro-Par Achievement Award 2015; the Spanish National Julio Rey Pastor award, in recognition of research in Mathematics; the Spanish National Award “Leonardo Torres Quevedo” that recognizes research in engineering; the “King Jaime I” in basic research given by Generalitat Valenciana; the Research Award by the Catalan Foundation for Research and Innovation and the “Aragón Award” 2008 given by the Government of Aragón. He has been named Honorary Doctor by the University of Chalmers, by the University of Belgrade, by the Universities of Las Palmas de Gran Canaria, Zaragoza, Complutense de Madrid and Cantabria in Spain and by the University of Veracruz in Mexico. "Hall of the Fame" member of the ICT European Program (selected as one of the 25 most influents European researchers in IT during the period 1983-2008. Lyon, November 2008)
In December 1994, Professor Valero became a founding member of the Royal Spanish Academy of Engineering. In 2005 he was elected Correspondant Academic of the Spanish Royal Academy of Science, in 2006 member of the Royal Spanish Academy of Doctors, in 2008 member of the Academia Europaea and in 2012 Correspondant Academic of the Mexican Academy of Sciences. He is a Fellow of the IEEE, Fellow of the ACM and an Intel Distinguished Research Fellow.
In 1998 he won a “Favourite Son” Award of his home town, Alfamén (Zaragoza) and in 2006, his native town of Alfamén named their Public College after him.
Abstract
Runtime Aware Architectures
In the last few years, the traditional ways to keep the increase of hardware performance to the rate predicted by the Moore's Law have vanished. When uni-cores were the norm, hardware design was decoupled from the software stack thanks to a well defined Instruction Set Architecture (ISA). This simple interface allowed developing applications without worrying too much about the underlying hardware, while hardware designers were able to aggressively exploit instruction-level parallelism (ILP) in superscalar processors. Current multi-cores are designed as simple symmetric multiprocessors (SMP) on a chip. However, we believe that this is not enough to overcome all the problems that multi-cores face. The runtime has to drive the design of future multi-cores to overcome the restrictions in terms of power, memory, programmability and resilience that multi-cores have. In this talk, we introduce a first approach towards a Runtime-Aware Architecture (RAA), a massively parallel architecture designed from the runtime's perspective.